-
Continual Representation Learning for Biometric Identification,
Bo Zhao, Shixiang Tang, Dapeng Chen, Hakan Bilen, Rui Zhao,
Winter Conference on Applications of Computer Vision (WACV), 2021.
-
Self-paced Contrastive Learning with Hybrid Memory for Domain Adaptive Object Re-ID,
Yixiao Ge, Feng Zhu, Dapeng Chen, Rui Zhao, Hongsheng Li,
Conference on Neural Information Processing Systems (NeurIPS), 2020.
-
Self-supervising Fine-grained Region Similarities for Large-scale Image Localization,
Yixiao Ge, Haibo Wang, Feng Zhu, Rui Zhao, Hongsheng Li,
European Conference on Computer Vision (ECCV), 2020.
-
RBF-Softmax: Learning Deep Representative Prototypes with Radial Basis Function Softmax,
Xiao Zhang, Rui Zhao, Yu Qiao, Hongsheng Li,
European Conference on Computer Vision (ECCV), 2020.
-
Structured Domain Adaptation for Unsupervised Person Re-identification,
Yixiao Ge, Feng Zhu, Rui Zhao, Hongsheng Li,
arXiv:2003.06650, 2020.
-
COCAS: A Large-Scale Clothes Changing Person Dataset for Re-identification,
Shijie Yu, Shihua Li, Dapeng Chen, Rui Zhao, Junjie Yan, Yu Qiao,
IEEE International Conference on Computer Vision and Pattern Recognition (CVPR), 2020. (Acceptance rate: 22%)
-
Density-Aware Feature Embedding for Face Clustering,
Senhui Guo, Jing Xu, Dapeng Chen, Chao Zhang, Xiaogang Wang, Rui Zhao
IEEE International Conference on Computer Vision and Pattern Recognition (CVPR), 2020. (Acceptance rate: 22%)
-
Learning to Cluster Faces via Confidence and Connectivity Estimation,
Lei Yang, Dapeng Chen, Xiaohang Zhan, Rui Zhao, Chen Change Loy, Dahua Lin
IEEE International Conference on Computer Vision and Pattern Recognition (CVPR), 2020. (Acceptance rate: 22%)
-
Memory-Based Neighbourhood Embedding for Visual Recognition,
Suichan Li, Dapeng Chen, Bin Liu, Nenghai Yu, Rui Zhao
IEEE International Conference on Computer Vision (ICCV), 2019. (Acceptance rate: 25%)
[PDF]
[Abstract]
Learning discriminative image feature embeddings is of great importance to visual recognition. To achieve better feature embeddings, most current methods focus on designing different network structures or loss functions, and the estimated feature embeddings are usually only related to the input images. In this paper, we propose Memory-based Neighbourhood Embedding (MNE) to enhance a general CNN feature by considering its neighbourhood. The method aims to solve two critical problems, ie, how to acquire more relevant neighbours in the network training and how to aggregate the neighbourhood information for a more discriminative embedding. We first augment an episodic memory module into the network, which can provide more relevant neighbours for both training and testing. Then the neighbours are organized in a tree graph with the target instance as the root node. The neighbourhood information is gradually aggregated to the root node in a bottom-up manner, and aggregation weights are supervised by the class relationships between the nodes. We apply MNE on image search and few shot learning tasks. Extensive ablation studies demonstrate the effectiveness of each component, and our method significantly outperforms the state-of-the-art approaches.

-
AdaScale: Adaptively Scaling Cosine Logits for Effectively Learning Deep Face Representations,
X. Zhang, R. Zhao, Y. Qiao, X. Wang , H. Li
IEEE International Conference on Computer Vision and Pattern Recognition (CVPR), 2019. (Acceptance rate: 25.2%)
[PDF]
[Abstract]
[Bibtex]
Coming soon.

@inproceedings{zhang2019p2sgrad,
title = {AdaScale: Adaptively Scaling Cosine Logits for Effectively Learning Deep Face Representations},
author={Zhang, Xiao and Zhao, Rui and Qiao, Yu and Wang, Xiaogang and Li, Hongsheng},
booktitle={CVPR},
year={2019}
}
-
P2SGrad: Refined Gradients for Optimizing Deep Face Models,
X. Zhang, R. Zhao, J. Yan, M. Gao , Y. Qiao, X. Wang, H. Li
IEEE International Conference on Computer Vision and Pattern Recognition (CVPR), 2019. (Acceptance rate: 25.2%)
[PDF]
[Abstract]
[Bibtex]
Coming soon.

@inproceedings{zhang2019p2sgrad,
title = {P2SGrad: Refined Gradients for Optimizing Deep Face Models},
author={Zhang, Xiao and Zhao, Rui and Yan, Junjie and Gao, Mengya and Qiao, Yu and Wang, Xiaogang and Li, Hongsheng},
booktitle={CVPR},
year={2019}
}
-
Attention-Aware Compositional Network for Person Re-identification,
J. Xu, R. Zhao, F. Zhu, H. Wang, W. Ouyang
IEEE International Conference on Computer Vision and Pattern Recognition (CVPR), 2018. (Acceptance rate: 28.4%)
[PDF]
[Abstract]
[Bibtex]
[Code]
[Supplementary Material]
[Poster]
[DOI]
Coming soon.

@inproceedings{xu2018attention,
title = {Attention-Aware Compositional Network for Person Re-identification},
author={Xu, Jing and Zhao, Rui and Zhu, Feng and Wang Huaming and Ouyang, Wanli},
booktitle={CVPR},
year={2018}
}
-
Crossing-line Crowd Counting with Two-phase Deep Neural Networks,
Z. Zhao, H, Li, R. Zhao, X. Wang
European Conference on Computer Vision (ECCV), 2016. (Acceptance rate: 28.4%)
[PDF]
[Abstract]
[Bibtex]
[Code]
[Supplementary Material]
[Poster]
[DOI]
In this paper, we propose a deep Convolutional Neural Network (CNN) for counting the number of people across a line-of-interest (LOI) in surveillance videos. It is a challenging problem and has many potential applications. Observing the limitations of temporal slices used by state-of-the-art LOI crowd counting methods, our proposed CNN directly estimates the crowd counts with pairs of video frames as inputs and is trained with pixel-level supervision maps. Such rich supervision information helps our CNN learn more discriminative feature representations. A two-phase training scheme is adopted, which decomposes the original counting problem into two easier sub-problems, estimating crowd density map and estimating crowd velocity map. Learning to solve the sub-problems provides a good initial point for our CNN model, which is then fine-tuned to solve the original counting problem. A new dataset with pedestrian trajectory annotations is introduced for evaluating LOI crowd counting methods and has more annotations than any existing one. Our extensive experiments show that our proposed method is robust to variations of crowd density, crowd velocity, and directions of the LOI, and outperforms state-of-the-art LOI counting methods.

@inproceedings{zhao2016crossline,
title = {Crossing-line Crowd Counting with Two-phase Deep Neural Networks},
author={Zhao, Zhuoyi and Li, Hongsheng and Zhao, Rui and Wang, Xiaogang},
booktitle={ECCV},
year={2016}
}
-
Saliency Detection by Multi-context Deep Learning,
R. Zhao, W. Ouyang, H. Li, X. Wang
IEEE International Conference on Computer Vision and Pattern Recognition (CVPR), 2015. (Acceptance rate: 28.4%)
[PDF]
[Abstract]
[Bibtex]
[Code]
[Supplementary Material]
[Poster]
[DOI]
Low-level saliency cues or priors do not produce good enough saliency detection results especially when the salient object presents in a low-contrast background with confusing visual appearance. This issue raises a serious problem for conventional approaches. In this paper, we tackle this problem by proposing a multi-context deep learning framework for salient object detection. We employ deep Convolutional Neural Networks to model saliency of objects in images. Global context and local context are both taken into account, and are jointly modeled in a unified multi-context deep learning framework.
To provide a better initialization for training the deep neural networks, we investigate different pre-training strategies, and a task-specific pre-training scheme is designed to make the multi-context modeling suited for saliency detection. Furthermore, recently proposed contemporary deep models in the ImageNet Image Classification Challenge are tested, and their effectiveness in saliency detection are investigated. Our approach is extensively evaluated on five public datasets, and experimental results show significant and consistent improvements over the state-of-the-art methods.
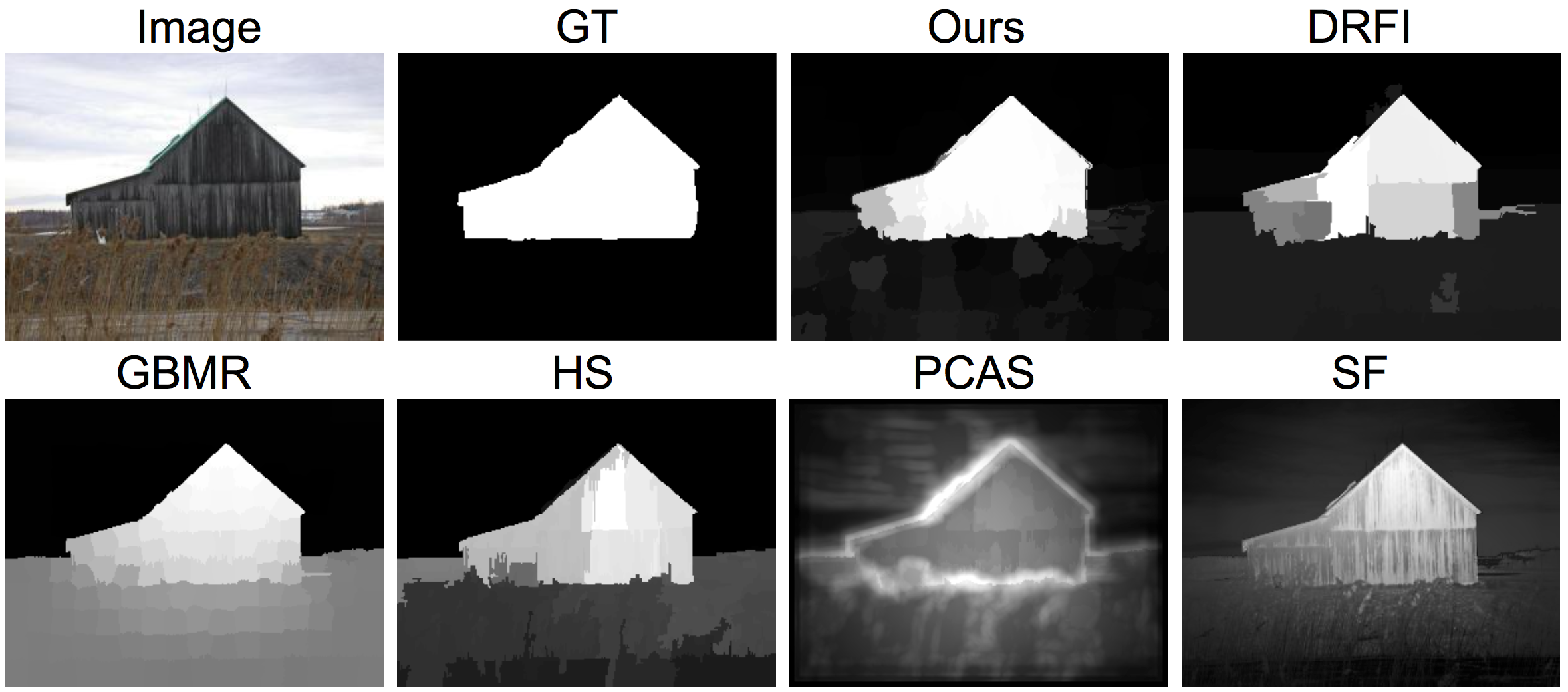
@inproceedings{zhao2015saliency,
title = {Saliency Detection by Multi-context Deep Learning},
author={Zhao, Rui and Ouyang, Wanli and Li, Hongsheng and Wang, Xiaogang},
booktitle={CVPR},
year={2015}
}
-
DeepReid: Deep Filter Pairing Neural Network for Person Re-Identification,
W. Li, R. Zhao, T. Xiao, X. Wang.
IEEE International Conference on Computer Vision and Pattern Recognition (CVPR), 2014. (Acceptance rate: 29.8%)
[PDF]
[Abstract]
[Bibtex]
[Poster]
[DOI]
Person re-identification is to match pedestrian images from disjoint camera views detected by pedestrian detectors. Challenges are presented in the form of complex variations of lightings, poses, viewpoints, blurring effects, image resolutions, camera settings, occlusions and background clutter across camera views. In addition, misalignment introduced by the pedestrian detector will affect most existing person re-identification methods that use manually cropped pedestrian images and assume perfect detection.
In this paper, we propose a novel filter pairing neural network (FPNN) to jointly handle misalignment, photometric and geometric transforms, occlusions and background clutter. All the key components are jointly optimized to maximize the strength of each component when cooperating with others. In contrast to existing works that use handcrafted features, our method automatically learns features optimal for the re-identification task from data. The learned filter pairs encode photometric transforms. Its deep architecture makes it possible to model a mixture of complex photometric and geometric transforms. We build the largest benchmark re-id dataset with 13,164 images of 1,360 pedestrians. Unlike existing datasets, which only provide manually cropped pedestrian images, our dataset provides automatically detected bounding boxes for evaluation close to practical applications. Our neural network significantly outperforms state-of-the-art methods on this dataset.
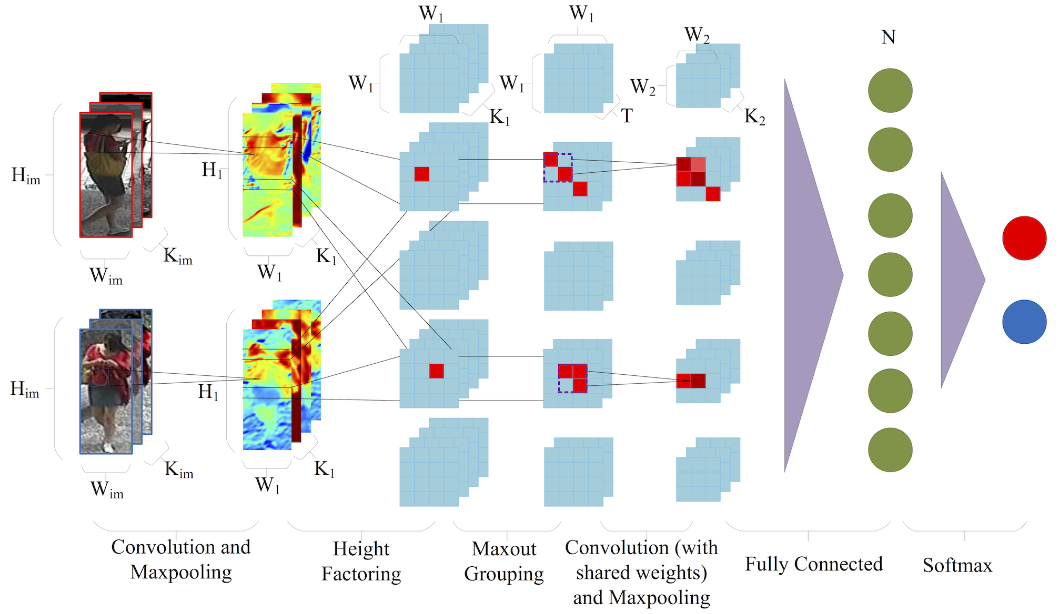
@inproceedings{li2014deepreid,
title = {DeepReid: Deep Filter Pairing Neural Network for Person Re-identification},
author={Li, Wei and Zhao, Rui and Xiao, Tong and Wang, Xiaogang},
booktitle = {IEEE Conference on Computer Vision and Pattern Recognition (CVPR)},
year = {2014},
month = {June},
address = {Columbus, USA}
}
-
Learning Mid-level Filters for Person Re-Identfiation,
R. Zhao, W. Ouyang, X. Wang.
IEEE International Conference on Computer Vision and Pattern Recognition (CVPR), 2014. (Acceptance rate: 29.8%)
[PDF]
[Abstract]
[Bibtex]
[Project Page]
[Poster]
[Code]
[DOI]
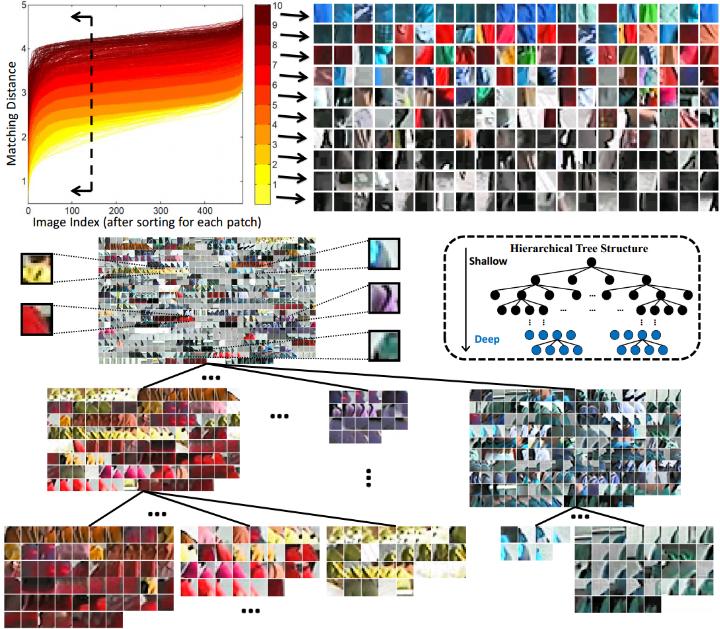 |
In this paper, we propose a novel approach of learning mid-level filters from automatically discovered patch clusters for person re-identification. It is well motivated by our study on what are good filters for person re-identification. Our mid-level filters are discriminatively learned for identifying specific visual patterns and distinguishing persons, and have good cross-view invariance. First, local patches are qualitatively measured and classified with their discriminative power. Discriminative and representative patches are collected for filter learning. Second, patch clusters with coherent appearance are obtained by pruning hierarchical clustering trees, and a simple but effective cross-view training strategy is proposed to learn filters that are view-invariant and discriminative. Third, filter responses are integrated with patch matching scores in RankSVM training. The effectiveness of our approach is validated on the VIPeR dataset and the CUHK01 dataset. The learned mid-level features are complementary to existing handcrafted low-level features, and improve the best Rank-1 matching rate on the VIPeR dataset by 14%.
|
@inproceedings{zhao2014learning,
title = {Learning Mid-level Filters for Person Re-identfiation},
author={Zhao, Rui and Ouyang, Wanli and Wang, Xiaogang},
booktitle = {IEEE Conference on Computer Vision and Pattern Recognition (CVPR)},
year = {2014},
month = {June},
address = {Columbus, USA}
}
-
Unsupervised Salience Learning for Person Re-Identification,
R. Zhao, W. Ouyang, X. Wang.
IEEE International Conference on Computer Vision and Pattern Recognition (CVPR), 2013. (Acceptance rate: 25.2%)
[PDF]
[Abstract]
[Bibtex]
[Project Page]
[Poster]
[Code]
[DOI]
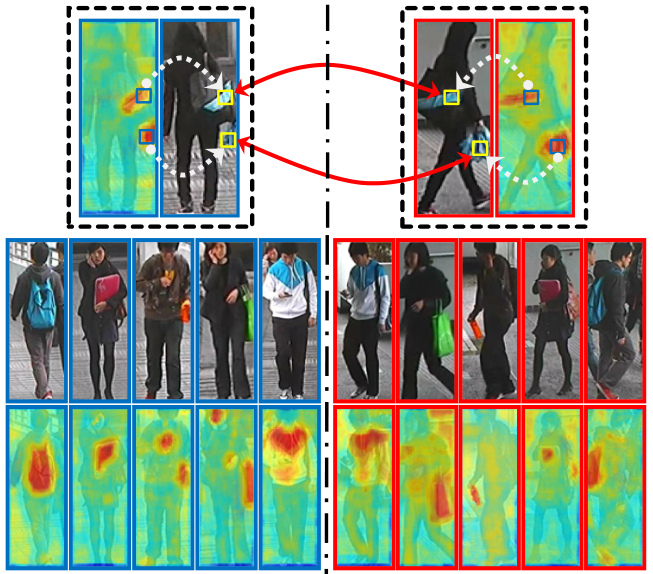 |
Human eyes can recognize person identities based on some small salient regions. However, such valuable salient information is often hidden when computing similarities of images with existing approaches. Moreover, many existing approaches learn discriminative features and handle drastic viewpoint change in a supervised way and require labeling new training data for a different pair of camera views. In this paper, we propose a novel perspective for person re-identification based on unsupervised salience learning. Distinctive features are extracted without requiring identity labels in the training procedure. First, we apply adjacency constrained patch matching to build dense correspondence between image pairs, which shows effectiveness in handling misalignment caused by large viewpoint and pose variations. Second, we learn human salience in an unsupervised manner. To improve the performance of person re-identification, human salience is incorporated in patch matching to find reliable and discriminative matched patches. The effectiveness of our approach is validated on the widely used VIPeR dataset and ETHZ dataset.
|
@inproceedings{zhao2013unsupervised,
title = {Unsupervised Salience Learning for Person Re-identification},
author={Zhao, Rui and Ouyang, Wanli and Wang, Xiaogang},
booktitle = {IEEE Conference on Computer Vision and Pattern Recognition (CVPR)},
year = {2013},
month = {June},
address = {Portland, USA}
}
-
Person Re-Identification by Salience Matching,
R. Zhao, W. Ouyang, X. Wang.
In Proceedings of IEEE International Conference on Computer Vision (ICCV), 2013. (Acceptance rate: 27.5%)
[PDF]
[Abstract]
[Bibtex]
[Project Page]
[Poster]
[Code]
[CMC]
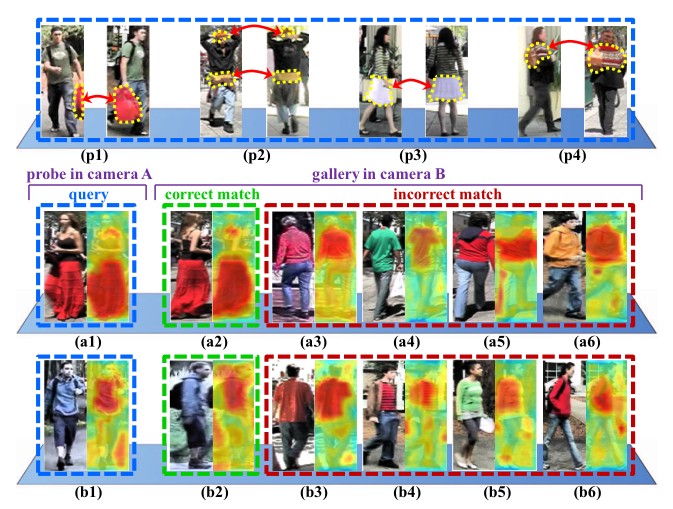 |
Human salience is distinctive and reliable information in matching pedestrians across disjoint camera views. In this paper, we exploit the pairwise salience distribution relationship between pedestrian images, and solve the person re-identification problem by proposing a salience matching strategy. To handle the misalignment problem in pedestrian images, patch matching is adopted and patch salience is estimated. Matching patches with inconsistent salience brings penalty. Images of the same person are recognized by minimizing thesalience matching cost. Furthermore, our salience matching is tightly integrated with patch matching in a unified structural RankSVM learning framework. The effectiveness of our approach is validated on the VIPeR dataset and the CUHK Campus dataset. It outperforms the state-of-the-art methods on both datasets.
|
@inproceedings{zhao2013person,
title = {Person Re-identification by Salience Matching},
author={Zhao, Rui and Ouyang, Wanli and Wang, Xiaogang},
booktitle = {IEEE International Conference on Computer Vision (ICCV)},
year = {2013},
month = {December},
address = {Sydney, Australia}
}
-
Human Reidentification with Transferred Metric Learning,
W. Li, R. Zhao, X. Wang.
In Proceedings of Asian Conference on Computer Vision (ACCV), 2012. (Oral Acceptance rate: 3.6%)
[PDF]
[Abstract]
[Bibtex]
[Dataset]
[DOI]
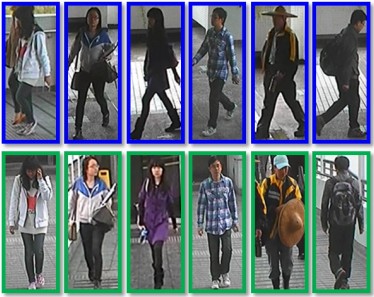 |
Human reidentification is to match persons observed in nonoverlapping camera views with visual features for inter-camera tracking. The ambiguity increases with the number of candidates to be distinguished. Simple temporal reasoning can simplify the problem by pruning the candidate set to be matched. Existing approaches adopt a fixed metric for matching all the subjects. Our approach is motivated by the insight that different visual metrics should be optimally learned for different candidate sets. We tackle this problem under a transfer learning framework. Given a large training set, the training samples are selected and reweighted according to their visual similarities with the query sample and its candidate set. A weighted maximum margin metric is online learned and transferred from a generic metric to a candidate-set-specific metric. The whole online reweighting and learning process takes less than two seconds per candidate set. Experiments on the VIPeR dataset and our dataset show that the proposed transferred metric learning significantly outperforms directly matching visual features or using a single generic metric learned from the whole training set.
|
@inproceedings{li2012human,
title = {Human Reidentification with Transferred Metric Learning},
author={Li, Wei and Zhao, Rui and Wang, Xiaogang},
booktitle = {Proceedings of Asian Conference on Computer Vision (ACCV)},
year = {2012}
}
-
SVD Based Linear Filtering in DCT Domain,
L. Zhuang, R. Zhao, N. Yu, B. Liu.
IEEE International Conference on Image Processing (ICIP), 2010.
[PDF]
[Abstract]
[Bibtex]
[DOI]
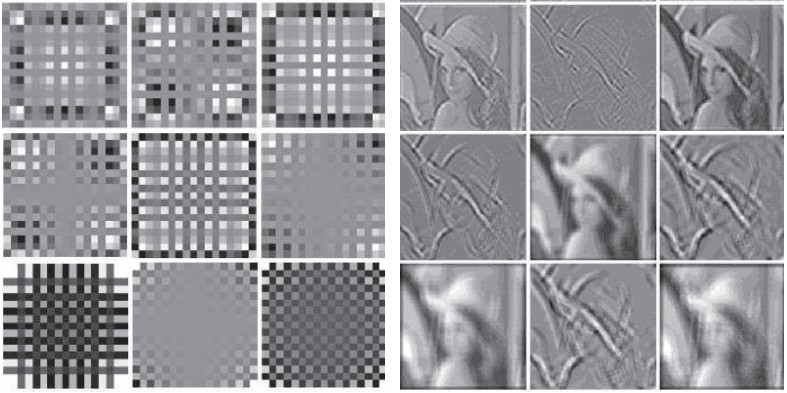 |
Efficient linear filtering in DCT domain is important in the area of processing and manipulation of image and video streams compressed in DCT-based method. In this paper, we proposed a novel method for linear filtering in DCT domain, regardless of filter type. We decompose any filter by SVD into weighted separable sub-filters which are well studied. Then we do fast linear filtering using these separable subfilters in DCT domain, and combine their results. To our best knowledge, it is the first method capable to do linear filtering with any type of filters directly in DCT domain. The scheme is demonstrated and discussed by doing Gabor filtering in DCT domain. Experiment results show that convolution result using the proposed solution is the same as that in spatial domain. Furthermore, our scheme is well suitable for distributed computing, which will improve computing speed greatly.
|
@inproceedings{zhuang2010SVD,
title = {SVD Based Linear Filtering in DCT Domain},
author={Zhuang, Liansheng and Zhao, Rui and Yu, Nenghai and Liu, Bin},
booktitle = {IEEE International Conference on Image Processing (ICIP)},
year = {2010}
}